Mobile Photographing and Searching System
Running into beautiful scenery, beautiful clothes, shoes in new style, blooming flowers, many of us would like to know more about them. However, not knowing the name of the location, the brand and the species of them makes it difficult to search for more information in the Internet. ¡®Mobile Terminal Photographing and Searching System¡¯ brings the wish into reality. Based on mobile terminal, it can satisfy the demand of searching anywhere at any time. Using the mobile terminals installed with the software of us, the users can take photo of the scenery, clothes, shoes, and then point out the interesting area in the photo. Then the users upload it to the Internet and the information of the photo (such as the brand of the shoes, the species of the flowers, etc.) will be returned together with many similar images. At present, our group is working on the searching system of shoes and plants, named ¡®Mobile Shoes Searching¡¯ and ¡®Wiki for Plants¡¯, respectively.
Medical image Retrieval System
The medical image retrieval system is developed for retrieving the images in hospital database. The retrieved images are expected to be relevant to the sign keywords or lesion regions that the user inputs. The system provides a good auxiliary tool for doctors to perform diagnosis, treatment, research and education of diseases in lung, colon, breast, and other anatomic site. The system runs on the user¡¯s work station and is connected with PACS (Pictures Archiving and Communication System) or other image storage systems from which the search results are returned.
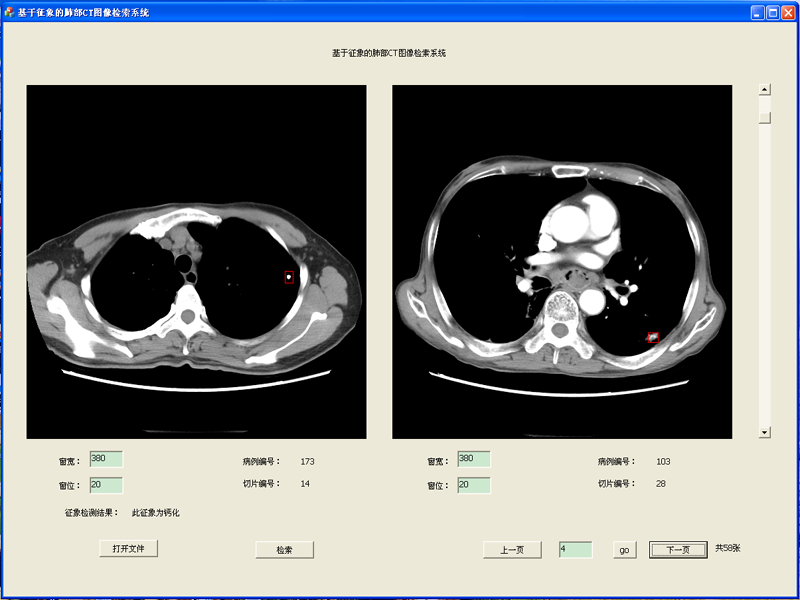
Lung CT Imaging Sign Database:
The well-characterized medical image database is very important for the development and evaluation of computer-aided diagnostic (CAD) methods. In fact, the medical image database is a common limitation for the development of CAD methods, because the task of identifying and collecting appropriate images is a laborious and challenging process. We are developing a lung manifestation database to meet the requirements for lesion detection and manifestation classification. Just like other researchers, we believe that an organized collection of clinical images alone would provide a valuable resource and would eliminate database composition as a source of variability that hinders the appropriate comparison of different CAD methods.[Top]
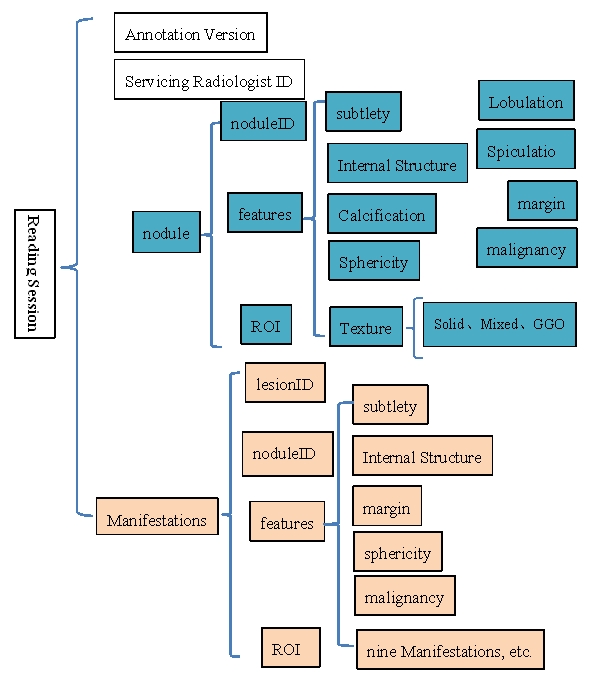
Interactive Image Segmentation:
The interactive image segmentation is different from a traditional automatic segmentation. The interactive image segmentation attempts to join user interaction in the segmentation process as input data of the algorithm, so that objects having a high-level semantic can be extracted out of the image. The interactive image segmentation algorithm which we have proposed is described as the following procedure. First of all, you only need to use a rectangle to mark the target object, then you will get the segmentation result. If you are not satisfied with the result, you can utilize secondary interaction button to carry on image segmentation twice or more times. You need to brush the outline of the object simply when doing the secondary interactions. Then the better segmentation result can be obtained. The effect diagram of the Interactive image segmentation is shown in the figure below.
Image Search Engine Improving:
The image search engine improvement is a part of our work, which analyzes and improves the retrieval results of image search engine. Type a keyword, such as ¡®basket¡¯, in your favorite Internet image search engine, say Google Image (or Bing, etc.). What do you get? In the thousands of images these search engines return, a great deal of irrelevant images are included. Based on these raw results, we do researches aiming at improving the accuracy of them. Our works take the raw results returned by image search engine as the training examples and a classifier related to the inputted keyword is trained. Then the obtained classifier is used to compute the relevance degrees between the images and the given query. The images are re-ranked in descending order of their relevance degrees and returned to the user. The improving process can help the user to find more relevant images early and thus reduce his labor for browsing the ranking in depth.Examples of top 10 images in raw Google search results and our improved results, where the red boxes indicate irrelevant images and the others are relevant; the leftmost are the query keywords: (a) raw Google results; (b) our improved results.
Video Retrieval System
We are interested in technology about video retrieval, It¡¯s one of the most excited topic in the area of video. Video Retrieval is based on Machine Learning and Pattern Recognition, included that Video Segmentation, Video feature extraction, Video Index building and Evaluation of the Video Retrieval System. Besides this, In order to deal with the magnanimity video data retrieval, we are also do some research about distributing retrieval. We intend to develop a video retrieval system.